The objective of this contribution is to present the latest technological developments in ultrasonography used in a clinical environment, which are being implemented in the state-of-the-art ultrasound devices supplied by leading manufacturers. It focuses on solutions dedicated to point-of-care ultrasonography (POCUS). It would be reasonable to ask whether artificial intelligence (AI) is able to replace humans also in this respect, and if so, to what extent. Not long ago, radiologists claimed that ultrasonography could not find any use in diagnostics of the respiratory system because ultrasounds dissipate fully in air, and hence also in aired lungs [1]. Such an approach was completely denied by Daniel Lichtenstein, whose literature pioneered lung ultra-sonography. He was also the author of such ultrasound protocols as BLUE (Bedside Lung Ultrasound in Emergency), FALLS (Fluid Administration Limited by Lung Sonography), SESAMEE (Sequential Echographic Scanning Assessing Mechanism), and Origin of Severe Shock of Indistinct Cause, as well as the LUCI-FLR (Lung Ultrasound Critically III Favouring Limitation of Radiation) project [2, 3]. The above protocols make therapeutic decisions easier for practitioners who are not radiologists, which appears crucial in cases of life saving decisions. When applied by a physician, such a protocol may and should be used to extend the available clinical context.
Throughout the last decade this diagnostic method gained popularity for use on a routine basis for bedside examination, especially in hospital emergency and rescue departments (ER) and intensive care units (ICU). Gradually POCUS has become an instrument used in Poland also in other medical disciplines, such as internal diseases, surgery, or paediatrics. It is now well established in anaesthesiology and intensive medical care as well as emergency medicine. Particularly in ultrasonography, application of this instrument for diagnostics of the respiratory system or haemodynamic monitoring became an integral part of practice of numerous physicians thanks to improved availability of ultrasound systems [4].
The progress observed in ultrasound diagnostics was the outcome of several important factors: rapid development of technology, access to state-of-the art equipment dedicated to POC, use of non-invasive procedures and consequently no significant complications, and wider spectrum of applications [5]. To meet expectations of the physicians, manufacturers design and develop even more innovative and highly helpful technical solutions based upon AI concept to assist the physician’s work in the environment of an operating theatre, ICU, hospital accident and ER, as well surgical, internal, and paediatric wards. Such solutions offered by different suppliers of medical equipment are, for example, iScanHelper, iVocal, eSpecialNavi, SmartVTI, SmartIVC, AutoEF, or Smart B-Line, named diffe-rently by individual manufacturers.
SELECTED NOVEL TECHNOLOGICAL SOLUTIONS IMPLEMENTING THE AI SCHEMES IN ULTRASOUND SYSTEMS
Learning the basic skills needed to perform the bedside POCUS examination demands about 20 to 50 procedures under the guidance of an instructor. The most common are procedures carried out at ICU as well as ER, including, e.g., qualitative evaluation of the heart or presence of fluid in the peritoneal cavity [6]. The number of innovative technological solutions implemented in ultrasound units, which may be referred to as AI, has been growing immensely over the recent few years. Among the first medical disciplines to implement AI was radiology. The innovative character of the solution assumed analysis by an advanced computer system of hundreds of thousands of stored X-ray images of selected fragments of the human body and comparing them to the present diagnostic images. The comparative analysis and evaluation of certain radiological features were presented in the form of a final report and description of the investigation. Such a solution allows for determination of the biological age of the patient, taking into consideration the so-called skeletal age [7]. The most interesting POCUS solutions developed in recent years include the following:
– iScanHelper (Mindray Medical International Limited, hereafter referred to as the Mindray). Using a touchscreen, iScanHelper presents a live image of the carried-out investigation along with images of anatomical and sono-anatomical patterns supplemented with a description. In addition, the latest systems often inform the user of whether the obtained image is optimal and suggest how to manipulate the ultrasound probe to change the angle of insonation and obtain an optimal image. Thanks to this solution, the measurements taken on the obtained image are highly reliable and repeatable, which is of critical importance for the POCUS concept. Originally, iScanHelper was used in regional anaesthetics, ensuring great support for practitioners performing the regional block. At present, the solution may also find its use when examining a patient at the ICU or ER, within the adopted POC protocols (Figure 1).
– iVocal (Mindray). Not only does the ultrasound unit ensure intuitive touch mode operation but also the option to control the system by giving voice commands. This may prove extremely important in enhanced sanitary regime conditions during such procedures as central line, regional block, or any other in which the operator’s contact with the ultrasound unit should be minimalised.
FIGURE 1
iScanHelper – educational software integrated with the ultrasound system (author’s own source)
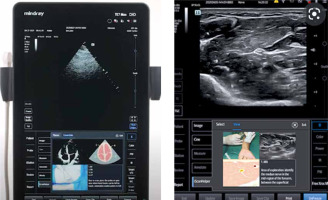
Ultrasound needle navigation or eSpecialNavi (Mindray) or Needle Assist (General Electric hereafter referred to as the GE) is a system which, similarly to car navigation, indicates to the operator performing the ultrasound-guided invasive procedure whether it is proceeding properly, and particularly if the needle remains within the ultrasound beam axis, the depth of the needle, or what move the head should make to visualise the needle on the screen. Thanks to the neodymium magnets in the ultrasound probe and the appropriate software, the system recognises the position of the needle against the ultrasound beam (Figure 2).
FIGURE 2
Graphic presentation of ultrasound navigation with software integrated with the ultrasound system (author’s own source)

– SmartVTI (Mindray) or Auto VTI (GE). Based on the left ventricular outflow tract (LVOT) and the manual measurement of the velocity time integral (VTI), the ultrasound software allows for evaluation of the stroke volume (SV) as well as the cardiac output (CO). SmartVTI ensures fully automatised measurement of VTI. Thanks to such software, once proper ultrasound projection (5-chamber, apical) is reached, the sampling gate of the Pulsed Wave (PW) Doppler is placed automatically along the left ventricle stroke route while the screen displays the trend graph indicating the VTI, SV, and CO. This ensures instant availability of data needed in the diagnostic process (Figure 3).
– AutoEF (Mindray/GE) is the system for automatic measurement of the left ventricular ejection fraction (LVEF), one of the most important parameters for the evaluation of cardiac efficiency. Similarly to SmartVTI, the measurement is fully automatic. This time, however, the only task of the operator is to obtain proper apical, 4-chamber or 2-chamber projection and then to click on the AutoEF icon to launch automatic measurement of the ejection fraction (EF). Following this, the system automatically outlines the systolic and diastolic left ventricle in real time. Making use of Simpson’s method (biplane disk summation), the system presents measurement of the end-diastolic volume (EDV) and EF. This ensures the objective character of the EF evaluation made by the operator [8]. The usefulness of such methods depends on the sonoanatomic condition of the patient (Figure 4).
– SmartIVC (Mindray) or AUTO IVC (GE) provides automatic measurement of the inferior vena cava, which helps to estimate susceptibility to fluid therapy. A condition to obtain a trend graph documenting changes in the collapsibility index and the diameter index (DI) is projection of the inferior vena cava along the long axis on the level of the xiphoid process. As soon as an image like that presented in Figure 5 is obtained, along with the fragment of the right atrial orifice, it is possible to click on the SmartIVC icon to start the automatic measurement. The system will automatically present collapsibility index and DI values in real time along with the quotient of the 2 parameters expressed as the collapsibility percentage.
FIGURE 3
Graphic presentation of SmartVTI software – automatic calculation of velocity time integral (VTI) (author’s own source)

FIGURE 4
Graphic presentation of AutoEF software – automatic fraction measurement (author’s own source)

FIGURE 5
Graphic presentation of Smart B-Line software – automatic measurement of the inferior vena cava (author’s own source)

It should be noted, however, that each measurement may be flawed due to mobility characteristics of the inferior vena cava, which may result in measurement of one of the chords and not the actual diameter of the vessel.
Among the presented modern and automatic technical solutions that find their use also beyond the departments of anaesthesiology, ICUs, and ERs, special attention should be devoted to Smart B-line (Mindray) and Auto B-line (GE) technologies. Both offer support to a physician performing ultrasound assessment of the respiratory system. Their main feature is automatic interpretation and calculation of the vertical artefacts, B-lines, and calculation of the percentage of B-lines in the given intercostal space to which the head has been applied. Detection of B-lines results in delivery of a detailed report with a visual map scoring areas of the lungs. One of the obstacles during the procedure itself and upon interpretation of the lung artefacts may be the left side of the patient’s body, which for obvious reasons is more difficult to interpret due to the anatomical position of the heart, restricting access to the intercostal spaces.
The state-of-the-art solutions described above are just some of the innovations in ultrasound technologies developed in the last few years. The question is whether AI implemented in medicine can replace a physician. Numerous authors who evaluate and implement AI in the world of medicine are convinced that its role is not to replace humans but merely to deliver instruments to significantly extend the scope of the bedside diagnostic and therapeutical process [7].
LUNG ULTRASONOGRAPHY AS INTERPRETATION OF ARTEFACTS, NOT SONO-ANATOMICAL STRUCTURES
Lung ultrasound has become one of the key elements of diagnostic imaging throughout ICUs as well as ERs, ensuring evaluation of both the lungs and the pleura. Bedside ultrasound systems have become more and more available, thus reducing traditional chest diagnostics based on X-ray and CT scanning, reducing the patient’s exposure to X-ray radiation [9, 10]. Ultrasound investigations of the lungs are based primarily upon thorough evaluation of the vertical and horizontal artefacts and their consolidation, which may prove the presence of different pathologies. Nevertheless, the analysis of artefacts should always be confronted with clinical information, such as the patient’s history, physical examination, or results of laboratory tests and microbiological investigations [11]. The lung ultrasound is an exceptional investigation because it is based on interpretation of the artefacts, not visualisation of the anatomical structure of organs on the ultrasound device screen [10]. The major vertical and horizontal artefacts to be interpreter are lines A, B, C, E, I, and Z. Evaluation of the artefacts, including B-lines, has practical use in the diagnostics and monitoring of emergency conditions as well as haemodynamic assessment of the patient. Their origin is complicated and not yet fully investigated [12]. Individual artefacts, symptoms, signs, and characteristic ultrasound images may be summarised as presented in the Table 1.
SMART B-LINE AS THE BASIC INSTRUMENT TO INTERPRET B-LINES IN THE ULTRASOUND IMAGE – THEORY AND PRACTICE
Ultrasound evaluation of the lung is a diagnostic imaging method comprising multiple areas of the lung. One of the elements of the ultrasound procedure is interpretation of B-lines [13]. Multiple contributions are available to assess the practical use of AI, not only in POCUS but throughout the medical disciplines. The subject of numerous studies carried-out in recent years was also the analysis of data delivered by AI for automatic calculation of B-lines. Evaluating the studies delivered throughout the last 4 years, and convinced of the justified use of AI, we observe how it is evolving towards common application in physicians’ daily practice.
One of the original studies, published in 2017, indicated that acquisition of skills needed to identify B-lines required a specialist training programme, while interpretation itself depended on the person performing such an investigation. It was also suggested that implementation of automatic B-line detection would benefit in improved assessment of lung aeration in both children and adults, including the adult dialysed patients. Based on the random method (mathematical method for calculation of blurred lines of different amplitude and phase used in imaging) [14], the authors concluded that the efficacy of such an automated method surpassed the traditional one even as much as 50% [15].
Another study during the same period showed that after performing about 25 ultrasound procedures supervised by experts, it was possible to achieve an acceptable level of competence, while legitimacy of automation of quantitative B-line assessment was still investigated. Moreover, it is unclear whether bedside ultrasound devices will ever replace a stethoscope in the practitioner’s hands [16].
Further publications described the use of ultrasound technology (BK3000 system) integrated with automatic detection and visualisation of B-lines, used to examine 4 healthy individuals and 4 patients after cardiosurgical procedures. The results demonstrated that the system was able to detect B-lines while the AI algorithm successfully differentiated between healthy individuals and those with pulmonary oedema. It was also shown that the system differentiated between diverse pulmonary areas where the oedema was not distributed in a uniform manner. Consequently, the authors confirm that the automated methods may potentially find their use in different clinical conditions [17].
Repeatability of B-line detection may also depend on the type of the ultrasound probe used for lung examination. While single, vertical artefacts should raise no doubts, their increased number may cause the human eye to be less reliable, not to mention the question of assessment of the distance between individual lines, which tend to consolidate as characteristic for the interstitial syndrome. Automatic methods for calculation of such characteristic lines prove very helpful because subjective evaluation by different operators is excluded here. Also, current recommendations point to the need for evaluation of the percentage of B-lines throughout the investigated region, which effects the subjective nature of such as procedure [18].
Deterioration of the epidemiological status due to the coronavirus pandemic also raised more interest in bedside evaluation of the lungs. Taking into consideration the organisational, logistic, epidemiological, and resource constraints in the defined quarantine zones, the question of evaluation of the lungs, in particular interpretation of the artefacts, became another challenge for the physicians. One of the studies carried out during COVID-19 pandemic, in the conditions of intensive medical care, at the third largest hospital in Israel – the Soroka University Medical Centre – efficiency of the automatic B-line detection system implemented in a Venue ultrasound system by GE was assessed in patients of the ward. Analysis of 153 dynamic images recorded during investigations showed the reliability of the automatic B-line detection system in patients with severe course of COVID-19. The following publication proves better interpretation of B-lines by an AI system in the investigated pulmonary regions as compared to the subjective “inter-operator” evaluation, i.e. performed by different operators. What is more, in future such solutions may find their use also in telemedicine, substantially reducing the duration of the procedure. The COVID-19 pandemic has clearly contributed to the development of such technologies [19]. The authors of another study, carried out in 2023 at Ben-Gurion University of the Negev and Soroka Medical Centre, Israel, concluded that the POCUS investigation is dependent on the operator, and the technologies they evaluated showed significant accordance between POCUS expert and AI technology. The effectivity ranged between 45% and 91% depending on the technology assessed. The highest accordance was observed in LVOT VTI while the lowest was seen in EF, which clearly illu-strates that such technologies may play a role in the progress of the POCUS learning process [20]. In 2020, when a pilot assessment of technology effectiveness was performed for automatic measurement of inferior vena cava collapse with the use of the EchoNous Inc. device, which was then entering the market, the result was merely moderate. On the other hand, the publication pointed to tremendous potential for its use in clinical practice [21]. Further development of AI technologies in quantitative and qualitative quantification was confirmed by another study describing investigations carried out in 2021 at the Faculty of Emergency Medicine, School of Medicine, Indiana University, where the technology was assessed based on 611 pulmonary regions, 87 scanning images, and 29 patients. The conclusions drawn illustrate that bedside evaluation of the lungs is technically not difficult to learn; however, interpretation of the obtained images may raise certain doubts. A group of 51 beginners attempted at quantitative evaluation of B-lines after just a 30-minute training programme. One of the elements of such evaluation was a comparison of the number of B-lines in patients with acute cardiac insufficiency with the use of an AI system implemented to the ultrasound device to interpretation of the same lines performed by experts. Most importantly, the beginners made use of AI solutions, while the experts relied on their visual assessment. The authors reached a conclusion pointing to correlation, from moderate to strong, between data gathered by the beginners using AI and the expert group. Moreover, it was concluded that the results were promising, common clinical applications would require further studies [22]. Enhanced effectivity and accuracy of the investigation due to the use of AI in lung ultrasound assessment was also confirmed by a publication in 2022 in which possible diagnostic potential was evaluated in a pilot study comprising paediatric patients aged 0 to 17 years. The study was carried out enrolling a population of 32 patients with median age 5.5 years. The ultrasound investigations were carried out by physicians showing little experience, while the procedure itself was preceded by a one-hour training programme in POCUS. The results proved highly promising because the sensitivity and specificity were evaluated at 80% and 96%, respectively. The greatest share of AI-assisted accuracy was observed in the evaluation of pneumonia in the investigated population [23].
An instrument based on one of the most interesting AI solutions in POCUS, namely Smart B-line technology, is therefore helpful in daily clinical practice (Figure 6). Based on the developed algorithm of supervised machine learning, the technology employs a concept in which it would seem that learning is not accomplished through different devices, instruments, or machines but thanks to the learning of the machines themselves [7, 24]. The practical use of such technology ensures objective evaluation. Smart B-line combines the supervised machine learning (ML) algorithm with the capture of characteristic traits of the image for automatic monitoring of B-lines in real time. What is more, it ensures the user’s interaction with the system and the ultrasound device itself. The input data analysed by Smart B-line upon standard evaluation of the lungs as well as the output data include automatic detection of the pleural line, detection of acoustic shadows of the ribs, automatic outlining, calculation of B-lines (if they meet criteria for B-lines), and calculation of distances between the lines and scoring, as illustrated below:
score 0 (N) – if 0 to 2 B-lines are detected,
score 1 (B1) – for 3 or more B-lines,
score 2 (B2) – once adjacent B-lines are observed,
score 3 (C) – if images characteristic for air bronchogram or tissue-like sign are identified.
Data presenting the quantity of B-lines interpreted by Smart B-line technology along with their characteristic traits are converted to produce a legible description coded with colours for each of the investigated regions, i.e. green stands for “normal” score, yellow marks B1 “moderate”, B2 is orange meaning “severe”, while the score C stands for “lung consolidation”.
FIGURE 6
Parameters displayed on the screen upon lung evaluation with the use of Smart B-Line technology (after www.mindray.com)

Artificial intelligence solutions used in ultrasound systems to calculate objectively the B-lines within the pulmonary regions show certain constraints; for example, those associated with the additional cost of Smart B-line software. It should also be noted that automatic detection is possible only if the operator is aware of the dynamic nature of the ultrasound procedure, which practically means that applying the head in different pulmonary regions may not result in detection of B-lines by AI. According to the principles of the reverberation effect and sound wave reflection, potential artefacts require the proper insonation angle during the investigations. The practical use of AI systems demands from the user at least the basic skills necessary to operate the ultrasound device, which must be preceded by theoretical and practical knowledge to use POCUS in lung evaluation.
CONCLUSIONS
Artificial intelligence has undoubtedly found its place in POCUS. However, like any technological innovation, it should be treated with a certain degree of caution. Although relatively easy to perform at the bedside, it should be noted that interpretation of the obtained imaged is highly subjective. The role of AI is to support the operator interpreting the visualised artefacts. It is certain that innovative solutions based on the machine learning algorithms for B-lines detection, like Smart B-line or automatic evaluation of the heart, SmartVTI, and SmartEF, as well as evaluation of the inferior vena cava collapse, SmartIVC, find extensive use in the clinical practice. Nevertheless, it is necessary to seek further improvements that would minimise the existing constraints. Artificial intelligence implemented to ultrasound systems will not replace physicians. However, it is likely to support them and assist in the decision-making process (Table 2).
TABLE 2
Summary of selected technological achievements used in POCUS (own source)
THE USE OF AI IN CLINICAL ULTRASONOGRAPHY
Although not all AI-based solutions were appropriately validated, e.g. by the FDA, they have already been widely implemented in clinical conditions [25]. A recent document issued by the FDA regarding the use of AI in medical devices states the following: “Artificial intelligence-based technologies have the potential to transform healthcare by deriving new and important insights from the vast amount of data generated during the delivery of healthcare every day”. COVID-19 pandemic accelerated the process of AI certification [26].
In 2020 the Northwestern Hospital in Chicago was among the first US hospitals to implement AI supporting physicians as well as less experienced physicians applying ultrasonography. The solutions were implemented in the ICU, ER, and the cardio-oncological clinic and used also by the hospital medicine group; in particular, AI found its application in the ICU conditions for bedside ultrasonography [27].
Similar clinical application of AI was accomplished by the Department of Anaesthesia and Intensive Care, Narayana Institute of Cardiac Sciences, Narayana Health City, Bangalore, India, wgere the technology was used to evaluate respiratory insufficiency, the inferior vena cava, or to calculate SV, CO, and EV in COVID-19 patients. Based on the daily clinical application of AI in that hospital, it was concluded that soon it would become an integral part of the daily routine in any hospital [23]. Moreover, extensive evaluation of clinical applications is now carried out by multiple international centres, such as Ben-Gurion University of the Negev, Beer Sheva 8410501, Israel; Soroka Medical Centre, Beer Sheva 84101, Israel; Department of Anaesthesiology and Intensive Care Medicine, Medical University Innsbruck, 6020 Innsbruck, Austria; or Ultrasound Laboratory Trento, University of Trento, Italy [20].