Purpose
Definitive concurrent chemoradiotherapy, comprised of external beam radiotherapy (EBRT) and chemotherapy followed by brachytherapy (BT), is the mainstay of treatment in locally advanced cervical cancer (LACC) [1, 2]. The first prospective multi-institutional observational cohort study using MRI-based brachytherapy in cervical cancer (EMBRACE-I) also achieved excellent long-term clinical outcomes, with few severe gastrointestinal and genitourinary morbidities [3]. Furthermore, in the era of 3D image-guided adaptive brachytherapy (3D-IGABT), the result of treatment was more favorable than 2D brachytherapy, especially when the advanced brachytherapy technique was applied [4-7]. Unfortunately, despite excellent local control of 92%, improvable pelvic control of 85% [3] still needs more powerful tools to differentiate the prognosis of the disease for further intensification of radiotherapy or additional treatments.
In recent decades, several nomograms have been developed to predict loco-regional recurrences and survival outcomes, but most were based on clinical factors alone. With the advancement of medical imaging and data science, radiomics was introduced to overcome human interpretation ability, especially to differentiate grayscale imaging affected by viewing angle, workstation luminance, and medical display capacity [8-11]. By combining radiomics with clinical data, better prediction models were reported for both LACC and early-stage cervical cancer [12-16]. Regarding radiomics of LACC treated with definitive radiotherapy, functional magnetic resonance imaging (MRI) and positron emission tomography and computed tomography (PET/CT) were investigated, and showed a prognostic value for the response, recurrence, survival, and radiation toxicity of the treatment [17-25]. However, limited use of these models was due to the lack of PET/CT in low socio-economic endemic area of LACC and non-specific mixture end points of recurrent and metastatic patterns reported in previous literature. With an increased accessibility [25, 26], magnetic resonance imaging became a potential modality for radiomics analysis in LACC.
Among the scarcity of MRI radiomics focusing on local and loco-regional prediction of recurrences [27-31], combined conventional and functional MRI, including diffusion-weighted image (DWI) and contrast-enhanced MRI, demonstrated better prediction compared with conventional sequence alone [31, 32]; however, technical heterogeneity limited image quality and interpretation, hindering the light of functional MRI. In addition, the mixture of neoadjuvant chemotherapy and 2D brachytherapy interfered with the interpretation of recurrence results and utility of the prognostic model in contemporary definitive concurrent chemoradiation, followed by the 3D-IGABT era.
Therefore, a single-sequence pre-treatment T2-weighted MRI radiomics for only patients who underwent 3D-IGABT was investigated for its loco-regional recurrence prognostic value, which can not only help to determine opportunities for radiotherapy and intensification of treatment, but also broaden the accessibility of radiomics in LACC.
Material and methods
Patient selection
A retrospective chart review was performed from January 2015 to June 2016. Patients were included if they had a histological diagnosis of stage IA to IVA cervical cancer according to the FIGO 2009 staging system, and received definitive radiotherapy or chemoradiotherapy with subsequent 3D-IGABT using MRI simulation before treatment. Patients were excluded if they had previous surgical treatment for cancer, including hysterectomy, artifact on MRI image in regions of interest, follow-up time of less than three months, incomplete clinical data, or a history of previous malignancy or metastatic disease. Clinical data were collected, including patient age, histological sub-type, hemoglobin level prior to initial treatment, FIGO 2009 staging, chemotherapy status, radiotherapy dose (including EBRT dose), BT dose at 90% of high-risk clinical target volume (D90 HR-CTV), and overall treatment time. Loco-regional recurrence events, response after three months of treatment, and living or dead status were included and analyzed.
MRI image acquisition protocol
All patients underwent pelvic MRI simulation using an abdominal coil with Philips Achieva 1.5-Tesla MR scanner on the same day of CT simulation. Before MRI examination, 20 ml of xylocaine jelly was inserted manually into the vagina. MR images were collected in 3D volumetric T2-weighted sequences with slice thickness of 1-2 mm. Field of view (FOV) was selected to cover all gross tumor extension. MRI parameters were repetition time (TR) = 2000 ms, TE = 120 ms, flip angle = 90°, matrix = 280 × 277, voxel size = 1 mm, and REC voxel size = 0.5 mm. MR images were reconstructed by routine protocol to show in axial planes, and were sent to EclipseTM software (Varian, a Siemens Healthineers Company, USA) in DICOM format images.
Imaging reading and feature extraction
After the contouring process, all axial MR images and gross tumor volume (GTV) structure were exported from EclipseTM software to DICOM files. In-house coding was written in MATLABR version 2016 platform, to separate GTV from the whole image. The segmented GTV was then used to extract radiomic characteristics from these DICOM files (Figure 1). Eighty radiomic features (Table 1) were extracted in GTV region using the in-house algorithm applied by MATLABR (Figure 1). Radiomic features included 20 features for first-order statistics, and 60 features for second-order statistics: 16 features for gray level co-occurrence matrix (GLCM) and 44 features for gray level run length matrix (GLRLM). For second-order statistics, a 32-level gray scale features extraction process was used, and 0, 45, 90, and 135 degrees of features in all axial planes were extracted. GLCM matrix was generated from GTV in each slice, then the first column and first row of the matrix to 0 were set to represent the background of images. For GLRLM matrix, the size of GLRLM matrix was adjusted before calculation. The radiomic feature ‘run percentage’ was employed to identify the total number of pixels in the matrix for calculation.
Fig. 1
Flow chart of the process of radiomics. A) MRI image segmentation; B) Feature extraction; C) Analysis
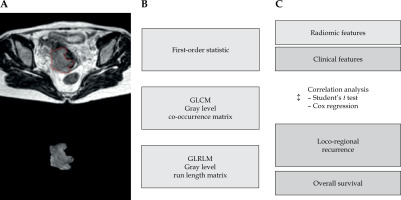
Table 1
Radiomic features
Feature selection and statistical analysis
There were many radiomic features, which could be relevant or irrelevant to clinical outcome. For a single hypothesis test, there is a small chance that type 1 error (false-positive) and type 2 error (false-negative) can occur, depending on an alpha level, typically 0.05 or 0.01. However, when multiple hypothesis testing is performed, the chance of obtaining a type 1 error can increase significantly. Therefore, a feature selection process was developed to minimize the use of statistical tests. The feature selection process was used to arrange radiomic features by impact on clinical outcome (Figure 1). Brown-Forsythe method was used to verify the equality of variance before univariate analysis, and Student’s t test was performed to compare between two groups, including the group with LRR and the group without LRR. The false discovery rate of the univariate analysis was controlled by Storey approach [33] that was chosen over Bonferroni method for its better statistical power and a lower false-negative rate [34]. A p-value of less than 0.01 was required for positive results. After the feature selection process, multivariate analysis was performed using Cox proportional hazards model that included significant radiomic features and clinical prognostic factors. The clinical prognostic factors were overall treatment time, hemoglobin level, HR-CTV dose, FIGO 2009 staging, chemotherapy use, and pelvic lymph node boost.
Results
Ninety patients treated between January 2015 and June 2016 were included. The clinical characteristics of patients are shown in Table 2. According to the initial FIGO 2009 staging, most patients were staged as IIIB (43 patients, 47.8%) and IIB (33 patients, 36.7%). Squamous cell carcinoma was found in most patients (67 patients, 74.4%), and adenocarcinoma in 17 patients (18.9%). The median dose of D90 HR-CTV was 86.2 Gy (IQR, 67.2-94.2). Most patients received concurrent chemoradiation (88.9%) with cisplatin or carboplatin. At a median follow-up time of 29.2 months, loco-regional recurrence (LRR) occurred in 12 (13.3%) patients and 19 (21.1%) patients died. Three months after completion of the treatment, six (6.7%) patients had progressive disease. The rate of 3-year loco-regional recurrence-free survival and overall survival was 85.9% and 83.1%, respectively (Figure 2).
Table 2
Patient characteristics
Fig. 2
Kaplan-Meier curves representing loco-regional recurrence-free survival (A) and overall survival (B)
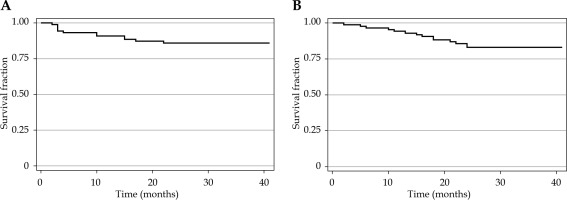
Eighty radiomic features were extracted. Seventeen radiomic features had p-values less than the false discovery rate (Supplementary Table 1). There were only four radiomic features that had a significant correlation with LRR: Max (p = 0.0002), Correlation135 (p = 0.0014), Correlation90 (p = 0.0015), and Correlation45 (p = 0.0034). The analysis of the Cox proportional hazards model with clinical factors demonstrated a statistically significant correlation with LRR (Table 3). However, other baseline characteristics, including concurrent chemoradiation, hemoglobin level, radiation dose, total treatment time, FIGO staging, and lymph node boost, were not significantly correlated with LRR (Table 4). A histogram of radiomics values of both the LRR group and the non-LRR group was explored, and there was no bi-modal distribution (Figure 3). There was no significant correlation of radiomic features with overall survival (Supplementary Table 2). We could not perform a statistical analysis using 3-month survival due to the small number of events. Each angle of radiomic feature in GLCM and GLRLM was strongly correlated. Using Pearson’s linear correlation method, coefficients of more than 0.85 between angles of each GLCM and GLRLM features were demonstrated (Supplementary Table 3).
Table 3
Radiomic features with loco-regional recurrence
Table 4
Baseline characteristics with loco-regional recurrence
Discussion
Radiomics has great potential to improve cancer patient care by predicting tumor response and treatment outcomes [34-38]. In our single-sequence pre-treatment T2-weighted MRI radiomics, maximum intensity and three correlations features were significant prognostic factors for LRR in the pre-treatment MRI for LACC patients treated with radiotherapy, followed by 3D-IGABT. In the Cox proportional hazard analysis, higher values of hazard ratios from radiomic features were associated with loco-regional recurrence compared with those from clinical factors. This finding is in line with finding from Zhang et al. [39] who evaluated 400 MRI radiomic features among 185 patients, and found that radiomic features had better predictive performance in progression-free survival than FIGO staging. For overall survival, there were no significant radiomic features found in this study, but each degree of second-order features provided similar values.
Park et al. [34] assessed pre-treatment MR radiomic features to pixel size re-sampling and interpolation in patients with cervical cancer, and reported that most first-order, shape, and texture features were robust after pixel size re-sampling and interpolation. Due to the high correlation between radiomic features, the Storey method was employed to reduce false discovery, but caution is still required to reduce type I and type II errors in multiple statistical tests [33].
In 2018, Lucia et al. reported a retrospective analysis of the radiomics of pre-treatment T2 MRI scans in 102 cervical cancer patients [20]. A total of 864 radiomic features were extracted from each sequence of pre-treatment PET/CT and MRI sequences composed of T2, diffusion-weighted imaging (DWI), and contrast enhancement (CE), and no significant association was found between radiomic features and local control or overall survival. A possible explanation is that the Bonferroni method was used instead of the Storey method for the false discovery control. In statistical analysis, using the Bonferroni method in numerous factors (875 factors) could yield high levels of type 2 error, especially if there are correlated factors [40, 41]. Moreover, in second-order features, Lucia et al. combined 13 degrees of matrix into one matrix before features extraction. In our study, we extracted each degree of matrix in the axial plane separately; therefore, the GLCM and GLRLM features between these two studies cannot be directly compared. Finally, the multi-modal follow-up imaging protocols were different between these two studies.
Lucia et al. investigated radiomic features from PET/CT and MRI for prognostic factors in cervical cancer treated with chemoradiotherapy. The authors demonstrated that entropy GLCM and GLNUGLRLM were independent predictors of recurrence and loco-regional control, with significantly higher prognostic power than usual clinical parameters. Additionally, they reported that entropy GLCM from functional imaging DWI-MRI and GLNUGLRLM from PET were independent predictors of recurrence [20]. However, these factors and other DWI-MRI-related radiomics that also showed significant correlation with local control, LRR, and survival in previous MRI-based radiomics [27, 32] were not investigated in our study to maintain the objective of single-sequence MRI radiomics utilization.
Developed exclusively on the conventional MRI sequence as our study, Kawahara et al. [30] established single and additional dual-sequence MRI radiomics consisting of not only T1 and T2 images from the standard tumor only volume of interest (VOI), but also the shrunk and expanded VOI that accounted for the intra- and peri-tumoral characteristics, leading to high accuracy of recurrence prediction in previous MRI radiomics [34]. The combined T1 and T2 models in Kawahara et al. study demonstrated excellent predictive performance superiority, with an area under the curve (AUC) of 0.94 compared with 0.89 and 0.69 of single-sequence T1 and T2 MRI radiomics, respectively. As the superiority of dual-sequence MRI radiomics did not accompany functional MRI, this would not compromise the intention of broad accessibility, but also enhance the power of conventional MRI radiomics. However, the inhomogeneity of 2D brachytherapy and 3D-IGABT may limit future utilization compared with homogeneous 3D-IGABT in the current study. In addition to pre-treatment imaging that account for the dynamic change during the course of radiotherapy, strong predictive power was demonstrated [27, 38], and should be considered as attractive information to be incorporated into conventional magnetic resonance radiomics. With a more precise transfer learning and transformation network [35, 37, 42], a further comparison study of conventional MRI integrating modified VOI and dynamic tumor change with or without incorporation of functional MRI radiomics in 3D-IGABT is awaited to reveal the non-inferiority. Furthermore, the shrinkage and expansion margin of tumor VOI should also be prospectively validated.
The main limitation of our study is its retrospective design. Although the pre-treatment MRI scans were performed on a single device, there was no complete record of MRI parameters, which could affect the quality of MRI. We explored the use of xylocaine gel as a quality control in MR images. The cylindrical geometry of the xylocaine gel (3 consecutive slices) was contoured in 10 patients, and first-order statistics were extracted. We found a large variability in intensity of values. For example, the maximum value in one case could be less than the minimum value in other cases. These could be affected by blood, vaginal discharge, or air bubbles in the vagina. Therefore, additional investigation is warranted by including a reference object when performing MRI for quality control.
A loco-regional recurrence rate of 13% was measured, while a study by Lucia et al. reported a 3-year recurrence rate of approximately 36% [20], and 23% recurrence rate was showed by Jajodia et al. [27] in the 3D brachytherapy era. This low proportion of recurrence may have limited our ability to test statistical significance and reliability of prognostic factors. A study with longer follow-up and larger sample size should increase the number of events and statistical power. Moreover, these two studies analyzed only the second-order radiomics, such as GLSZM features and GLDM features, entropy GLCM, and GLNUGLRLM, while the first-order radiomics were also analyzed and a significant correlation with clinical outcomes in this study was found. Apart from predicting clinical outcomes after CCRT, multi-time-point radiomics was also employed to predict tumor response during treatment. Meng et al. analyzed both the first-order and second-order radiomics, and found that ADC histogram shape analysis held the potential in monitoring early tumor response during CCRT [43]. This concept of radiomics analysis based on several time-points should be further evaluated for more robust outcomes in the future research. The comparison of outcomes from this study and relative studies is showed in Table 5.
Table 5
Comparison of outcomes from this study and relative studies
Finally, we used several methods and input parameters to extract an individual radiomic feature. For example, either the gray level scale or degree in GLCM or GLRLM features could be selected, and these two parameters would affect the result of the feature. By allowing different parameters in the feature extraction, some radiomic features could not be directly compared across the study. In the contouring process, the selection of the primary tumor and lymph node target as well as the delineation of the tumor edge could affect the outcome [34], especially in lymph node-positive disease and small tumor volumes. Therefore, it is important to assess the contouring process, input parameters, and extraction method to achieve a more precise interpretation.
In conclusion, we observed a significant correlation of single-sequence pre-treatment T2-weighted MRI radiomics with loco-regional recurrence in LACC patients treated with definitive radiotherapy and 3D-IGABT. However, further prospective investigations controlling image acquisition techniques, VOI modifications, dynamic tumor change monitoring, and follow-up schemes are needed to provide additional statistical power to confirm the utility of these conventional MRI radiomic features, for the benefit of universal accessibility and real-world utilization.